I'm a PhD on Face Presentation Attack Detection and right now I am working in Alice Biometrics on improving the technology of KYC automated solutions.
Eductation 📚
- B.Sc. in Telecommunication Engineering (Sound and Image) | University of Vigo (2010)
- Master in Multimedia Technologies | University of Granada (2012)
- Master in Computer Vision | University Rey Juan Carlos, Madrid (2013)
- Executive Master in Innovation | EOI, Escuela Organización Industrial (2013)
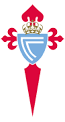
Face recognition technology is now mature enough to reach commercial products, such as smart phones or tablets. However, it still needs to increase robustness against imposter attacks. In this regard, face Presentation Attack Detection (face-PAD) is a key component in providing trustable facial access to digital devices. Despite the success of several face-PAD works in publicly available datasets, most of them fail to reach the market, revealing the lack of evaluation frameworks that represent realistic settings. Here, an extensive analysis of the generalisation problem in face-PAD is provided, jointly with an evaluation strategy based on the aggregation of most publicly available datasets and a set of novel protocols to cover the most realistic settings, including a novel demographic bias analysis. Besides, a new fine-grained categorisation of presentation attacks and instruments is provided, enabling higher flexibility in assessing the generalisation of different algorithms under a common framework. As a result, GRAD-GPAD v2, a comprehensive and modular framework is presented to evaluate the performance of face-PAD approaches in realistic settings, enabling accountability and fair comparison of most face-PAD approaches in the literature.
A face presentation attack detection (face-PAD) system is in charge of determining whether a face corresponds to a presentation attack or not. The vast majority of proposed solutions consider a static scenario, where models are trained and evaluated in datasets where all types of attacks and conditions are known beforehand. However, in a real-world scenario, the situation is very different. There, for instance, the types of attacks change over time, with new impersonation situations appearing for which little training data is available. In this paper we propose to tackle these problems presenting for the first time a continual learning framework for PAD. We introduce a continual meta-learning PAD solution that can be trained on new attack scenarios, following the continual few-shot learning paradigm, where the model uses only a small number of training samples. We also provide a thorough experimental evaluation using the GRAD-GPAD benchmark. Our results confirm the benefits of applying a continual meta-learning model to the real-world PAD scenario. Interestingly, the accuracy of our solution, which is continuously trained, where data from new attacks arrive sequentially, is capable of recovering the accuracy achieved by a traditional solution that has all the data from all possible attacks from the beginning. In addition, our experiments show that when these traditional PAD solutions are trained on new attacks, using a standard fine-tuning process, they suffer from catastrophic forgetting while our model does not.
Face recognition has achieved unprecedented results, surpassing human capabilities in certain scenarios. However, these automatic solutions are not ready for production because they can be easily fooled by simple identity impersonation attacks. And although much effort has been devoted to develop face anti-spoofing models, their generalization capacity still remains a challenge in real scenarios. In this paper, we introduce a novel approach that reformulates the Generalized Presentation Attack Detection (GPAD) problem from an anomaly detection perspective. Technically, a deep metric learning model is proposed, where a triplet focal loss is used as a regularization for a novel loss coined “metric-softmax”, which is in charge of guiding the learning process towards more discriminative feature representations in an embedding space. Finally, we demonstrate the benefits of our deep anomaly detection architecture, by introducing a few-shot a posteriori probability estimation that does not need any classifier to be trained on the learned features. We conduct extensive experiments using the GRAD-GPAD framework that provides the largest aggregated dataset for face GPAD. Results confirm that our approach is able to outperform all the state-of-the-art methods by a considerable margin.
Over the past few years, Presentation Attack Detection (PAD) has become a fundamental part of facial recognition systems. Although much effort has been devoted to anti-spoofing research, generalization in real scenarios remains a challenge. In this paper we present a new open-source evaluation framework to study the generalization capacity of face Presentation Attack Detection methods, coined here as face-GPAD. This framework facilitates the creation of new protocols focused on the generalization problem and sets fair procedures of evaluation and comparison between PAD solutions. We also introduce a large aggregated and categorized dataset to address the problem of incompatibility between publicly available datasets. Finally, we propose a benchmark adding two novel evaluation protocols: one for measuring the effect introduced by the variations in face resolution, and the second for evaluating the influence of adversarial operating conditions.
In the current context of digital transformation, the increasing trend in the use of personal devices for accessing online services has fostered the necessity of secure cyberphysical solutions. Biometric technologies for mobile devices, and face recognition specifically, have emerged as a secure and convenient approach. However, such a mobile scenario also brings some specific threats, and spoofing attack detection is, without any doubt, one of the most challenging. Although much effort has been devoted in anti-spoofing techniques over the past few years, there are still many challenges to be solved when implementing these systems in real use cases. This chapter analyses some of the gaps between research and real scenario deployments, including generalisation, usability, and performance. More specifically, we will focus on how to select and configure an algorithm for real scenario deployments, paying special attention to use cases involving limited processing capacity devices (e.g., mobile devices), and we will present a publicly available evaluation framework for this purpose.
In recent years, software-based face presentation attack detection (PAD) methods have seen a great progress. However, most existing schemes are not able to generalize well in more realistic conditions. The objective of this competition is to evaluate and compare the generalization performances of mobile face PAD techniques under some real-world variations, including unseen input sensors, presentation attack instruments (PAI) and illumination conditions, on a larger scale OULU-NPU dataset using its standard evaluation protocols and metrics. Thirteen teams from academic and industrial institutions across the world participated in this competition. This time typical liveness detection based on physiological signs of life was totally discarded. Instead, every submitted system relies practically on some sort of feature representation extracted from the face and/or background regions using hand-crafted, learned or hybrid descriptors. Interesting results and findings are presented and discussed in this paper.
For face authentication to become widespread on mobile devices, robust countermeasures must be developed for face presentation-attack detection (PAD). Existing databases for evaluating face-PAD methods do not capture the specific characteristics of mobile devices. We introduce a new database, REPLAY-MOBILE, for this purpose.1 This publicly available database includes 1,200 videos corresponding to 40 clients. Besides the genuine videos, the database contains a variety of presentation-attacks. The database also provides three non- overlapping sets for training, validating and testing classifiers for the face-PAD problem. This will help researchers in comparing new approaches to existing algorithms in a standardized fashion. For this purpose, we also provide baseline results with state- of-the-art approaches based on image quality analysis and face texture analysis.
Automatic face recognition in unconstrained environments is a challenging task. To test current trends in face recognition algorithms, we organized an evaluation on face recognition in mobile environment. This paper presents the results of 8 different participants using two verification metrics. Most submitted algorithms rely on one or more of three types of features: local binary patterns, Gabor wavelet responses including Gabor phases, and color information. The best results are obtained from UNILJ-ALP, which fused several image representations and feature types, and UC-HU, which learns optimal features with a convolutional neural network. Additionally, we assess the usability of the algorithms in mobile devices with limited resources.